Challenges of today’s drug development process
The pharmaceutical industry is facing unprecedented challenges to its current business model. Independent observers and industry analysts have also predicted its forthcoming significant decline (1). It is obvious that certain changes are long overdue if this industry is to meet investors’ expectation and at the same time come up with viable answers to unmet medical needs, i.e. successful treatments of currently incurable diseases.
Large pharmaceutical companies will need to invent new approaches and methodologies just in order to maintain their present status. First, they have to decide on what areas they should focus on, given their unique capabilities and strategic assets. Second, in order to profitably develop and market drugs they will need to explore new areas of science and include targeted customer groups at the early phase of a drug R&D process. Third, upon a decision what is their main focus, they will need to identify all the key capabilities necessary to achieve their goals, and to build or retain specific capacities that will give them significant advantages over their competition. They will also need to figure out where they can profitably add value for patients beyond just providing drugs. But, to obtain all this they will subsequently have to restructure their organizations to speed up the overall decision-making process, increase accountability and reduce overall cost (2).
All these changes will lead to the introduction of new market segments, besides the currently most explored market segment of treatment. The newly evolving market opportunities are mainly concentrated around following market segments – prevention, prediction, patient’s participation and personalization of medical approach and therapies. However, we should always bear in mind the ever-present and very important market segment of a drug follow-up. This trend has been already announced more than ten years ago by key analysts, but was never seriously considered so far (Figure 1).
Figure 1. Significant new market opportunities were opening up which expanded the role of the large pharmaceutical companies in the healthcare chain (modified from the last PricewaterhouseCoopers (PwC) business report from the 20th century).
Implementation of this approach in routine work practice was a major challenge for the drug discovery industry over the last decade. However, the exploration of new development opportunities is a must for today’s pharmaceutical businesses, since this represents a key element in the overall R&D cycle time reduction and resource preservation.
Improvement of R&D process
Reductions in R&D cycle time can be achieved in many ways. However, in less predictable systems, such as pharmaceutical R&D, approaches to cycle time reduction cannot be simply adopted as in predictable systems like production, although principles behind these methodologies have already been applied to some extent in drug manufacturing systems (3).
Considerations for reducing the cycle time of each phase of drug discovery and development are quite often project and phase specific. Each phase of development consists of a collection of unit processes, or individual tasks that consume time and resources. The sequential arrangement of these tasks can often be unique to an individual research project, exhibiting a significant variability introduced by the type of the science associated with every R&D project. Because of this inherent variability, cycle time reduction at a macro level can be achieved through task- and project-specific interventions aimed at reducing non-value added tasks and lag-times associated with completion of value added tasks. The role of an experienced project manager, assuming the commitment of his/hers project team, is crucial for the successful and timely completion of pre-defined milestones along an R&D process.
There are few key approaches identified for the reduction of project-specific cycle times, but the most important one is the portfolio selection. When selecting the R&D projects portfolio, the consideration of overall project cycle time should be also an instrument for reducing overall costs. For example, a cycle time of pivotal clinical trials is often a function of the disease state or a pursued indication itself.
The common approach is to reduce the attrition rate at advanced pre-clinical or clinical phases of drug development. This can be achieved by detecting as early as possible all the key factors that could be the reasons for withdrawals from market because of unmet safety or efficacy specifications. In this respect, predictive techniques and approaches are of great help for pharmaceutical scientists.
Predictive tools in early R&D process phases
In general, the efficacy of the candidate drugs can be unsatisfactory because of many reasons, but some factors are easier to avoid than the others. In general, poor oral bioavailability and pharmacokinetic properties are among the most cited reasons for stopping a drug development process. In addition, toxicity issues, which were not predicted by in vitro modeling as well low efficacies observed in later animal pharmacology models, are often reasons for eliminations of a lead compound development. Preclinical ADMET (absorption, distribution, metabolism, excretion and toxicity) studies can help in increasing attrition rates in early clinical phases Phase I (healthy volunteers, mainly safety studies) and Phase IIa (preliminary efficacy studies on a small number of patients) (4).
Given their highly specific target-binding characteristics, fully human or humanized monoclonal antibodies have greatly reduced off-target toxicity compared to small molecules (5). Similar approach to reduce the attrition rate in later phases is to develop targeted therapies for validated targets.
A search for a cost-effective approach that can predict toxicological liabilities or other undesirable ADME (absorption, distribution, metabolism, excretion) properties during the pre-clinical phase (especially for small chemical molecules, molecular weight ≤ 500 Da) is a paramount for all pharmaceutical companies as this would be a clear breakthrough in reducing the downstream attrition. All major pharmaceutical companies are pursuing this holy grail of the drug development (6). In fact, the number (and percentage) of drug candidates successfully entering Phase I has increased recently (7), in part due to a better preclinical characterization with improved ADMET properties and because of the use of wisely designed pharmaco-dynamics models during the early phases of drug development (8). Modeling and predicting pharmacological properties of molecules, like solubility, toxicity or blood-brain-barrier penetration is extremely important in both drug design and drug development. Modeling and prediction can improve our understanding of chemical and physical mechanisms behind these key properties and provide tools for predicting their values for molecules that are not yet synthesized. The group of scientists at Institute is developing new methods for predicting ADMET properties (9-11). Their project started as a collaboration with a pharmaceutical industry and it is worth mentioning that it‘s results were so valuable because of a carefully planned and performed in vitro and in vivoexperiments that provided the relevant and accurate data. This data was later used for testing and validation of developed mathematical models and prediction tools.
Predictive approach in advanced R&D phases
Going further toward the late stages of drug development, Phase II and Phase III attrition rates remain unacceptably high: 52% and 29%, respectively, based on the most recent benchmarking estimates (Figure 2) (12). Phase II attrition rate in particular have not improved substantially since those reported for the 1991–2000 period. At that time 62% attrition rate was reported for the Phase II and 45% for the Phase III. However, there are certain therapeutic areas that exhibit even higher attrition rates like neurology and gastroenterology (13).
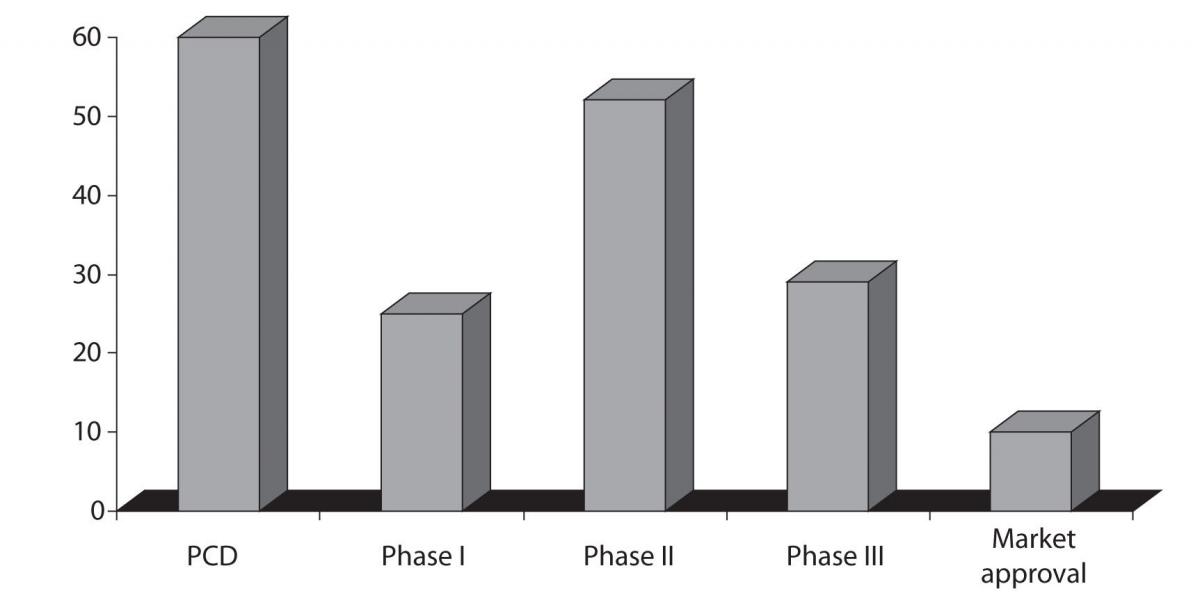
Figure 2. Chances of failure, attrition rates, during the drug development process; source: The Trufts Center for drug Development, data is representing the trends in 2008. Preclinical development - PCD
There are two key approaches to reduce Phase II and III attrition, which should ideally be carried out jointly (as noted by a number of authors) (14):
- the first is a better target selection (identification of validated and “druggable” targets);
- the second is a routine pursuit of early “proof of concept” clinical studies, especially in Phase I, for which biomarkers and surrogate endpoints can often be employed.
Early target selection and identification of first promising hits, combining in silico and biological screening is certainly one way for carrying out initial research for the new chemical entities in today’s pharmaceutical industry (15). This method synergistically combines synthetic chemistry, in vitro screening and computational drug design approaches in order to provide added value to compounds already existing in the depository or compounds bank of research institutes associated to pharmaceutical industry (16,17).
To optimize biomarker development and capture value, it is an imperative to ensure that biomarkers are developed early and that they are commercially available at the time of the drug launch (18).
One way to reduce development costs is to tailor therapies to specific patient population, as illustrated by the development of imatinib (Gleevec; Novartis) (19) or to stratify patient populations for both clinical development and commercialization, as in trastuzumab example (Herceptin; Genentech/Roche) (20).
It was predicted that advances in genomics and proteomics, including those resulting directly from the sequencing of the human genome, would yield an abundance of drug targets (21). However, although many new potential drug targets have been identified by these approaches, far too few have been sufficiently validated so far to have any impact on the pharmaceutical R&D. Nevertheless, we believe that in the very near future a use of validated drug discovery targets will be the way to succeed.
In this respect, it is crucial to strengthen the link between genomics, proteomics, biochemistry and the clinics in order to predict, as soon as possible, pharmacological response (22).
In addition, as a conclusion, enabling a wide range of synergies through connection of research groups from the educational, clinical and pharmaceutical sectors by establishing translational medicine centers will encourage translational research and translational medicine implementation. In this way, interaction of highly educated research staff, research support services, specialized experts in a multidisciplinary, trans-disciplinary and interdisciplinary environment will have a significant impact on improvement of general health and the higher quality of life.
Acknowledgements
I wish to thank Dr. Damir Nadramija for constructive and helpful suggestions during revision of the manuscript.
Notes
Potential conflict of interest
None declared.
References
1. Garnier J. Rebuilding the R&D engine in big pharmaceutical industries. Harvard Bus Rev 2008;86:68-76.
3. Pyzdek T, eds. The six sigma handbook: The complete guide for greebelts, blackbelts, and managers of all levels. 2nd ed. McGraw-Hill, 2003.
4. Paul SM, Mytelka DS, Dunwiddie CT, Persinger CC, Munos BH, Lindborg SR, et al. Schacht AL. How to improve R&D productivity: the pharmaceutical industry’s grand challenge. Nat Rev Drug Discov 2010;9:203-14.
5. Tan DS, Thomas GV, Garrett MD, Banerji U, de Bono JS, Kaye SB, et al. Biomarker-driven early clinical trials in oncology: a paradigm shift in drug development. Cancer J 2009;15:406-20.
6. Nigsch F, Macaluso NJ, Mitchell JB, Zmuidinavicius D. Computational toxicology: an overview of the sources of data and of modelling methods. Expert Opin Drug Metab Toxicol 2009;5:1-14.
8. Rohatagi S, Kastrissios H, Gao Y, Zhang N, Xu J, Moberly J, et al. Predictive population pharmacokinetic/pharmacodynamic model for a novel COX-2 inhibitor. J Clin Pharmacol 2007;47:358-70.
9. Lučić B, Nadramija D, Bašic I, Miličević A, Trinajstić N, Suzuki T, et al. Correlation of liquid viscosity with molecular structure for organic compounds using different variable selection methods. Arkivoc 2002;4:45-59.
10. Lucic B, Nadramija D, Basic I, Trinajstic N. Toward Generating Simpler QSAR Models. J Chem Inf Comput Sci 2003;43:1094-102.
11. Lučić B, Nadramija D. Modeling water solubility of molecules by ensemble of multivariate regression models. In: Aki-Sener E, Yalcin I, eds. The Euro-QSAR Proceeding Book. Istambul: 2004. p. 349.
13. Kola I, Landis J. Can the pharmaceutical industry reduce attrition rates? Nature Rev Drug Discov 2004;3:711-5.
14. Booth B, Zemmil R. Prospects for productivity. Nature Rev Drug Discov 2004; 3:451-6.
15. Verbanac D, Jelic D, Stepanic V, Tatic I, Ziher D, Kostrun S. Combined in silico and in vitro approach to drug screening. Croat Chem Acta 2005;78:133-9.
16. Jelic D, Mildner B, Koctrun S, Nujic K, Verbanac D, Culic O, et al. Homology modeling of human fyn kinase structure: discovery of rosmarinic acid as a new fyn kinase inhibitor and in silico study of its possible binding modes. J Med Chem 2007;22:1090-100.
17. Jelic D, Nujic K, Stepanic V, Kovacevic K, Verbanac D. 6-imino-2-thioxo-pyrimidinones as a new class of dipeptidyl peptidase IV inhibitors. Med Chem Res 2010 (in press).
18. Tan DS, Thomas GV, Garrett MD, Banerji U, de Bono JS, Kaye SB, Workman P. Biomarker-driven early clinical trials in oncology: a paradigm shift in drug development. Cancer J 2009;15:406-20.
19. Manley PW, Cowan-Jacob SW, Buchdunger E, Fabbro D, Fendrich G, Furet P, et al. Imatinib: a selective tyrosine kinase inhibitor. Eur J Cancer 2002;38:19-27.
20. Slamon DJ, Leyland-Jones B, Shak S, Fuchs H, Paton V, Bajamonde A, et al. Use of chemotherapy plus a monoclonal antibody against HER2 for metastatic breast cancer that overexpresses HER2. N Engl J Med 2001;344:783–92.
21. Grenet O. Significance of the human genome sequence to drug discovery. Pharmacogenomics J 2001;1:11–2.
22. Lippi G, Favaloro EJ. The missing link between genotype, phenotype and clinics. Biochem Med 2009;19:137-45.